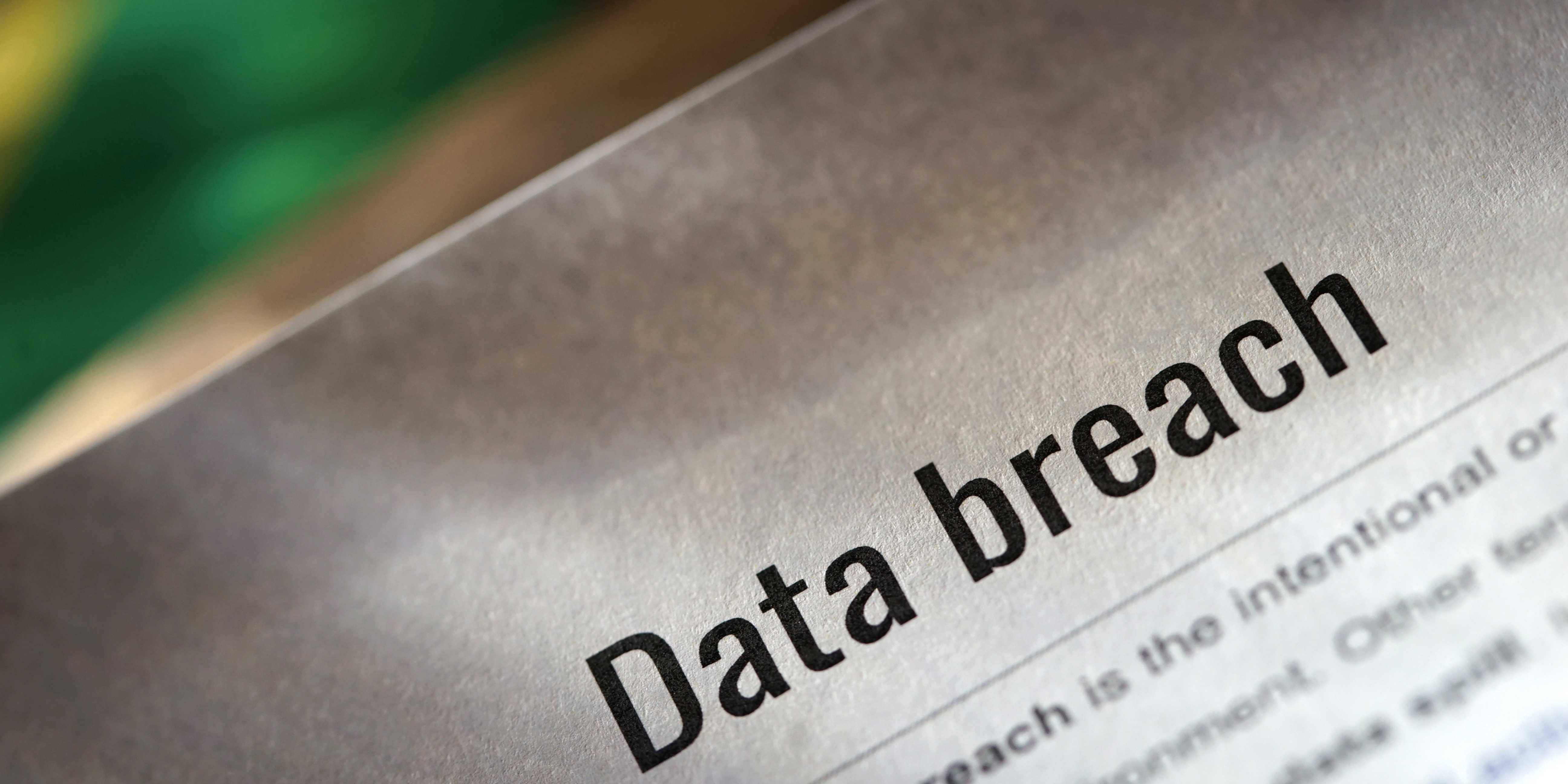
Privacy Breach Reporting Requirements under Law25
The evolving landscape of data protection in Canada sees provinces enacting or refining their respective privacy legislations to address the
Sometimes we take a break from building cutting edge AI redaction models to stretch our academic muscles and write about privacy and machine learning.
Don’t miss the latest on AI and privacy. Join our newsletter
The evolving landscape of data protection in Canada sees provinces enacting or refining their respective privacy legislations to address the
At Private AI, we pride ourselves on making scalable solutions for large companies, small businesses, and government institutions that need
Generative AI has become a cornerstone of modern technology, with potential applications ranging from content creation and customer service to
Webinar: Data Privacy in Healthcare Friday, April 19th – 1pm EST Sign up now Privacy Attacks against Data and AI
Webinar: Data Privacy in Healthcare Friday, April 19th – 1pm EST Sign up now Risks of Noncompliance and Challenges around
Webinar: Data Privacy in Healthcare Friday, April 19th – 1pm EST Sign up now Benefits of AI in Healthcare and
Introduction Data lakes are messy with vast amounts of data scattered everywhere as they serve as repositories for a myriad
About a year ago we covered the 2022 Cost of a Data Breach Report by IBM. The 2022 report had
In today’s data-driven landscape, the use of AI-based solutions, such as ChatGPT, has become increasingly prevalent. These solutions leverage the
Hello, dear community! We continue to see exciting improvements released since 3.7. Here is a synopsis of highlights from the
Data leaks are a serious threat to any business, and particularly those handling sensitive personal information, such as customer’s financial
New York State has taken a step towards ensuring responsible and ethical use of Artificial Intelligence (AI) technologies in the
Let’s say you need to run sentiment analysis on a dataset of reviews. You will use one of the pre-trained
South Korea’s Personal Information Protection Act was substantially revised in March of 2023. In addition, an Enforcement Decree was announced
In mid-January 2024, Australia’s Minister for Industry and Science, Ed Husic, unveiled the government’s response to the Safe and Responsible
On March 13, the European Parliament crossed another and (almost) the last hurdle for the EU AI Act to come
In the age of rampant AI development, data protection laws are no longer a tick-box exercise but a cornerstone of
We asked ChatGPT five privacy questions covering the GDPR, HIPAA, and US and Canadian private sector privacy laws and evaluated
Although they hardly need an introduction, Salesforce is a cloud-based customer relationship management (CRM) service that allows businesses to efficiently
Embeddings are an increasingly popular data science tool, used across the industry for various solutions. What are Embeddings? Embeddings essentially
We are excited to share the details of our latest platform update, which brings significant enhancements across various domains. Enhancing
After a political deal had been reached on December 8, 2023, we now have an unofficial draft of the EU
The California Consumer Privacy Act (CCPA) has been in effect since January 1, 2020, and it has impacted the way
In the US, there is, as of yet, no comprehensive federal privacy legislation, but there is a federal agency, the
On December 8, 2023, the European Parliament, European Commission, and Council of the EU reached a significant political agreement concerning
In the contemporary landscape of health information technology, the Health Insurance Portability and Accountability Act (HIPAA) Privacy Rule sets a
The European Union’s recent review of Canada’s data protection adequacy, a follow-up to the initial adequacy decision made in 2001,
Artificial Intelligence (AI) has infiltrated various sectors of our lives, leading to an urgent need for standardized frameworks to ensure
Recent updates to OpenAI’s privacy policies, terms of use applicable to individuals, and business terms have sparked the interest of
OpenAI is an organization known for developing a variety of artificial intelligence (AI) models and tools, which are accessible through
On December 20, the Quebec government published the Draft Regulation respecting the anonymization of personal information in the Quebec Gazette.
Hello, dear community! We are thrilled to announce Private AI’s final release of 2023 with Version 3.6. Let’s explore what’s
Leia em português aqui The Lei Geral de Proteção de Dados (LGPD), Brazil’s answer to data privacy, determines the rules
The realm of generative AI, encompassing technologies that generate content like text, images, and videos, have seen a significant surge
Data security and integrity are two critical concepts in the world of cybersecurity. While they are often discussed together, they
In the era of digital transformation, Large Language Models (LLMs) have emerged as powerful tools for businesses, enabling them to
67% of government agencies have increased their financial commitment to digital transformation. Long lines and endless paper documents no longer suffice
Quebec’s commitment to modernizing its data protection measures is evident in the provisions of Law25, the most important provisions of
Hello, dear community! We are thrilled to announce the release of Version 3.5. Packed with new features, improvements, and fixes
This blog has an accompanying Jupyter Notebook! Access the notebook Large Language Models (LLMs) like Azure’s OpenAI service have become
In the first part of this blog series, we discussed data privacy in Germany and the various obstacles associated with
The Biden-Harris Administration recently enacted a sweeping Executive Order to forge America’s path in responsible AI development, encouraging both innovation
The recently amended EU AI Act proposal we introduced in this blog post, would regulate “foundational models,” defined in Art.
In the field of artificial intelligence, Large Language Models (LLMs) such as GPT-4 stand out as a major innovation, proving
LangChain is a powerful tool that allows you to setup a conversation with your favourite LLMs with ease. If you’re worried
In the digital transformation era, Large Language Models (LLMs) like ChatGPT are increasingly being integrated into organizational workflows to boost
How Are Governments Using Generative AI? Generative AI has found a multitude of use cases across industries. As of now,
Hello, dear community! We are thrilled to announce the release of version 3.4 of our product. This release isn’t just
Ethical AI in Government Government agencies face a unique challenge when it comes to regulating AI while simultaneously using it.
What is Responsible AI? The promise of AI extends far beyond convenience. The technology holds the potential to revolutionize government
Explore the key differences and similarities between Quebec’s Law25 and the European Union’s GDPR in this in-depth comparison.
Explore the key differences and similarities between Quebec’s Law25 and the European Union’s GDPR in this in-depth comparison.
Canada’s federal Personal Information Protection and Electronic Documents Act (PIPEDA) has been a benchmark for businesses in managing personal information.
In recent years, the rise of artificial intelligence (AI) and machine learning technologies has ushered in a new era of
In recent years, the emergence of artificial intelligence (AI) and machine learning technologies has opened up a world of possibilities
Leveraging ChatGPT in healthcare presents numerous opportunities for improving patient care, streamlining administrative tasks, enhancing medical education, and more. However,
There are two types of fines under the GDPR with different monetary thresholds. The first are administrative fines under Art.
The revised Data Protection Act (DPA) in Switzerland is set to come into effect on September 1, 2023. The aim
India’s Digital Personal Data Protection Bill, 2023, a landmark legislation that aims to protect the privacy rights of Indian citizens
Personally identifiable information (PII) is any data that can be used to identify an individual directly or indirectly, such as
When organizations think about how to collect, use, and disclose personal data in compliance with privacy laws, the first thing
New Zealand updated its data protection law with the Privacy Act 2020, which came into force on December 1, 2020.
Australia has been considering a reform of its 1988 Privacy Act for several years now. While important amendments have been
This article outlines the proposed EU AI Act including the most recent amendments to the proposal. We cover in particular
In response to growing consumer demand for privacy, Apple introduced App Tracking Transparency (ATT) as a feature in 2021, enabling
With OpenAI’s and Azure OpenAI’s API offerings, businesses are enabled to develop their own applications on top of the powerful
The rapid advancement of generative artificial intelligence (AI) has raised intriguing questions about the copyrightability of AI-generated output. As AI
If you are building or thinking about using an application based on OpenAI’s ChatGPT or another large language model (LLM)
Bill C-27, which includes the proposed Consumer Privacy Protection Act (“CPPA”), the Personal Information and Data Protection Tribunal Act and
Healthcare organizations are required to perform a delicate balancing act between healthcare data protection and disclosure of high utility data
Privacy and ethics concerns around ChatGPT make the news every week, whether it’s the temporary ban of ChatGPT in Italy,
If your organization ever finds itself in the position of wishing or having to disclose personally identifiable information (PII), e.g.,
PHI stands for “Protected Health Information” and can include information about an individual such as blood type, condition, injury, etc.
PCI is often mentioned in the triage PII, PHI and PCI in the context of data protection. PCI stands for
ChatGPT took the world by storm, and companies everywhere are leveraging the OpenAI tool to streamline their processes, improve productivity,
With data privacy becoming an increasingly hot topic as major data breaches make headlines around the globe, the biggest question
This guide is for you if you already know what Law25 is and have read some of the other excellent
In this blog post, we examine the two types of Personal Identifiers: direct and quasi- (or indirect) identifiers, why we
Data privacy is becoming an increasingly important topic in an increasingly digitized world where powerful technologies are more and more
In an increasingly digital world where customer data is being collected at various touchpoints, the protection of personal information is
The latest data breaches are a regular topic in the news. Raising awareness about the prevalence and severity of the
Data protection is a critical concern in today’s digital world. As more and more data are collected and processed, the
On February 8, 2023, the International Organization for Standardization adopted privacy by design in ISO 31700:2023 as a voluntary standard
What does privacy mean to us at Private AI? As a tech company whose purpose it is to enhance privacy,
Large language models (LLMs) are a type of machine learning model that are trained on vast amounts of text data
In today’s data-driven world, businesses are constantly collecting information from their customers in order to provide a better product or
The Canadian healthcare and health tech space is robust and growing at warp speed. Globally health tech, especially in the AI
Sign Up What is the Metaverse? Before we get to privacy in the Metaverse, we first have to define “Metaverse”
Differential privacy is a hot topic given the many conflicting opinions on its effectiveness. For some background, we previously wrote
Over the years, large pre-trained language models like BERT and Roberta have led to significant improvements in natural language understanding
At Private AI, we are building a privacy suite centered around personally identifiable information (PII) detection and remediation in unstructured data, such as
In the previous episode of Private AI’s ML Speaker Series, Patricia Thaine (CEO of Private AI) sat down with Dr.
In the previous episode of Private AI’s ML Speaker Series, Patricia Thaine (CEO of Private AI) sat down with Dr.
In today’s world, large models with billions of parameters trained on terabytes of datasets have become the norm as language
There are several resources available on the internet on how to scale your Kubernetes pods based on CPU, but when
In the previous episode of Private AI’s ML Speaker Series, Patricia Thaine (CEO of Private AI) sat down with Arvid Frydenlund
Personally Identifiable Information (PII) is any data that can be used to identify an individual. This can be done using
Previously on Private AI’s speaker series CEO, Patricia Thaine, sat down with Franziska Boenisch to discuss her latest paper, ‘When the Curious Abandon Honesty: Federated
In the latest episode of Private AI’s ML Speaker Series, Patricia Thaine (CEO of Private AI) sits down to chat about
With the ever-growing number of global regulations, legislations, and amendments, it can be overwhelming to know where to start (or
Fill out the form below and we’ll send you a free API key for 500 calls (approx. 50k words). No commitment, no credit card required!
Expand the categories below to see which languages are included within each language pack.
Note: English capabilities are automatically included within the Enterprise pricing tier.
French
Spanish
Portuguese
Arabic
Hebrew
Persian (Farsi)
Swahili
French
German
Italian
Portuguese
Russian
Spanish
Ukrainian
Belarusian
Bulgarian
Catalan
Croatian
Czech
Danish
Dutch
Estonian
Finnish
Greek
Hungarian
Icelandic
Latvian
Lithuanian
Luxembourgish
Polish
Romanian
Slovak
Slovenian
Swedish
Turkish
Hindi
Korean
Tagalog
Bengali
Burmese
Indonesian
Khmer
Japanese
Malay
Moldovan
Norwegian (Bokmål)
Punjabi
Tamil
Thai
Vietnamese
Mandarin (simplified)
Arabic
Belarusian
Bengali
Bulgarian
Burmese
Catalan
Croatian
Czech
Danish
Dutch
Estonian
Finnish
French
German
Greek
Hebrew
Hindi
Hungarian
Icelandic
Indonesian
Italian
Japanese
Khmer
Korean
Latvian
Lithuanian
Luxembourgish
Malay
Mandarin (simplified)
Moldovan
Norwegian (Bokmål)
Persian (Farsi)
Polish
Portuguese
Punjabi
Romanian
Russian
Slovak
Slovenian
Spanish
Swahili
Swedish
Tagalog
Tamil
Thai
Turkish
Ukrainian
Vietnamese
Testé sur un ensemble de données composé de données conversationnelles désordonnées contenant des informations de santé sensibles. Téléchargez notre livre blanc pour plus de détails, ainsi que nos performances en termes d’exactitude et de score F1, ou contactez-nous pour obtenir une copie du code d’évaluation.
Number quoted is the number of PII words missed as a fraction of total number of words. Computed on a 268 thousand word internal test dataset, comprising data from over 50 different sources, including web scrapes, emails and ASR transcripts.
Please contact us for a copy of the code used to compute these metrics, try it yourself here, or download our whitepaper.